Plenary Talks
Detection and Classification of Acoustic Scenes and Events: Research Problems, Applications, and Methods
Tuesday, September 18, 10:00 – 11:00
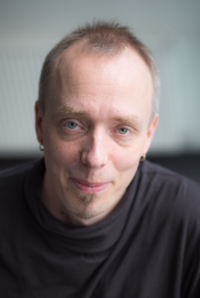
Prof. Tuomas Virtanen
Tampere University of Technology, Finland
Chair: Hiroshi Sawada
Abstract: Computational methods for detecting and classifying environmental sounds have recently been subject to significant amount of research. They have lots of potential applications, for example related to context-aware devices, acoustic monitoring, and multimedia information retrieval. There are also several scientific challenges related to the development of methods. This presentation will give an overview of the field, by discussing different tasks addressed, discussing various scientific and practical problems, and introducing some potential applications. It will present specific methods based on convolutional and recurrent deep neural networks, that have been used successfully in various tasks to obtain good results. The talk will also introduce the series of international DCASE evaluation campaigns, giving an overview of the tasks of the challenges and summary of results.
Download presentationBiography: Tuomas Virtanen is Professor at Laboratory of Signal Processing, Tampere University of Technology (TUT), Finland, where he is leading the Audio Research Group. He received the M.Sc. and Doctor of Science degrees in information technology from TUT in 2001 and 2006, respectively. He has also been working as a research associate at Cambridge University Engineering Department, UK. He is known for his pioneering work on single-channel sound source separation using non-negative matrix factorization based techniques, and their application to noise-robust speech recognition and music content analysis. Recently he has done significant contributions to sound event detection in everyday environments. In addition to the above topics, his research interests include content analysis of audio signals in general and machine learning. He has authored more than 150 scientific publications on the above topics, which have been cited more than 6000 times. He has received the IEEE Signal Processing Society 2012 best paper award for his article "Monaural Sound Source Separation by Nonnegative Matrix Factorization with Temporal Continuity and Sparseness Criteria" as well as three other best paper awards. He is an IEEE Senior Member, member of the Audio and Acoustic Signal Processing Technical Committee of IEEE Signal Processing Society, Associate Editor of IEEE/ACM Transaction on Audio, Speech, and Language Processing, and recipient of the ERC 2014 Starting Grant.
Machine Learning from Weak Supervision — Towards Accurate Classification with Low Labeling Costs
Wednesday, September 19, 9:30 – 10:30
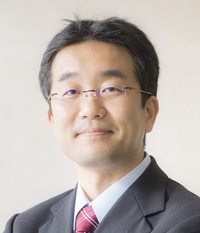
Prof. Masashi Sugiyama
RIKEN Center for Advanced Intelligence Project and The University of Tokyo, Japan
Chair: Hiroshi Saruwatari
Abstract:
Machine learning from big data is highly successful in real-world applications including image, speech, and natural language processing.
However, there are still various domains where the use of massive labeled data is prohibited.
In this talk, I will introduce our recent advances in machine learning from weak supervision, which is aimed at training an accurate classifier from training data collected with lower labeling costs than fully labeled data.
Examples include classification from only positive and unlabeled data, only positive-confidence data, and multi-class data with incorrect labels.
Finally I will briefly introduce the activities of RIKEN Center for Advanced Intelligence Project, which is a 10-year government-supported project covering theory and application of machine learning research and discussion of ethical and legal issues.
Biography: Masashi Sugiyama received the PhD degree in Computer Science from Tokyo Institute of Technology in 2001. After experiencing Assistant Professor and Associate Professor at the same institute, and he was appointed as Professor at the University of Tokyo in 2014. Since 2016, he has concurrently served as Director of RIKEN Center for Advanced Intelligence Project. He (co)authored several monographs including "Statistical Reinforcement Learning" (Chapman and Hall, 2015), "Density Ratio Estimation in Machine Learning" (Cambridge University Press, 2012), and "Machine Learning in Non-Stationary Environments" (MIT Press, 2012).
Machine Hearing in the Integrative Era: Connecting the Dots between Modalities and Tasks
Thursday, September 20, 9:30 – 10:30
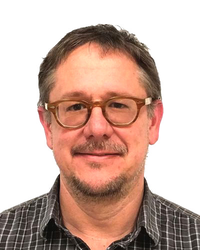
Dr. John Hershey
Google, U.S.A.
Chair: Tomohiro Nakatani
Abstract: To perceive the world around us in a coherent way requires integration of multiple percepts across multiple modalities. The past has been marked by a divergence of methods across modalities and tasks, with integration often left as an afterthought. However, with everything, from microphone array signal processing to audio-visual scene understanding, utilizing similar deep learning methods, we have now entered an era where integrative tasks are the first-class subjects of end-to-end modeling efforts. Long-standing issues for integration, such as robust fusion of multiple inputs, and the question of the correspondence between percepts across modalities, are resurfacing to be addressed by new approaches from the deep learning toolbox. This talk will present recent attempts to integrate different combinations of beamforming, source separation, visual processing, and multi-lingual speech recognition. Experimental work will be presented on a variety of integrative tasks that attempt to push the envelope of what can be done within a single coherent system. We are at a point in time where such work raises as many questions as it answers; the talk will highlight open issues and new directions suggested by the current state of the art.
Biography: John is a researcher at Google in Cambridge, Massachusetts where he leads a research team in machine perception, since joining in January 2018. Prior to that he spent seven years leading the speech and audio research team at MERL (Mitsubishi Electric Research Labs), and five years at IBM's T. J. Watson Research Center in New York, where he led a team of researchers in noise-robust speech recognition. He also spent a year as a visiting researcher in the speech group at Microsoft Research in 2004, after obtaining his Ph D from UCSD. Over the years he has contributed to more than 100 publications and over 30 patents in the areas of machine perception, speech processing, speech recognition, and natural language understanding.